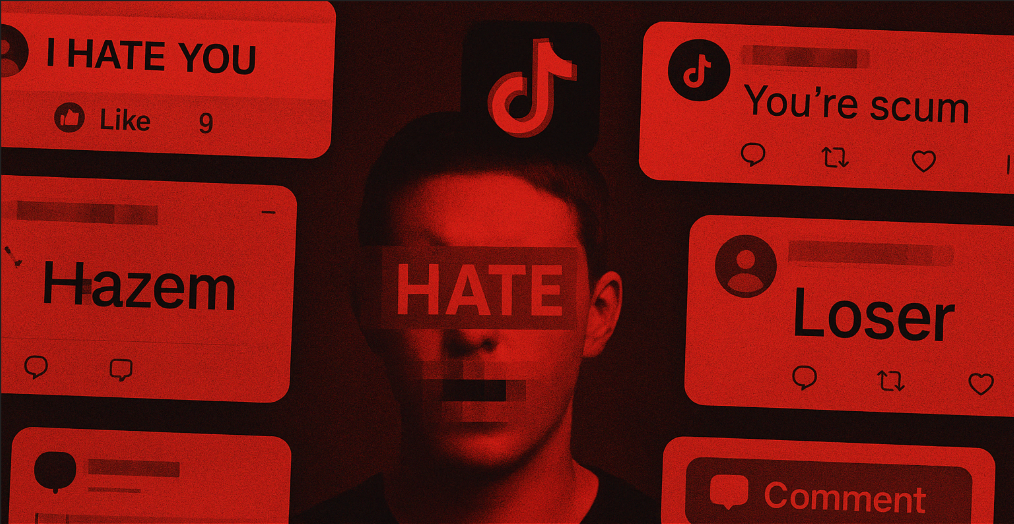
Hate Is Trending – And So Are the Profit
Have you ever noticed that the angrier the post, the higher it climbs in your feed? That extreme content seems to spread faster than anything else? That’s no accident. That’s the algorithm at work.
In Myanmar, a viral Facebook post comparing Rohingya Muslims to “cockroaches” was shared tens of thousands of times before offline mobs torched villages and mosques. In Sri Lanka, a rumor claiming Muslims poisoned food in Buddhist restaurants triggered mass violence after a Facebook post exploded in reach. In India, YouTube channels using titles like “Muslim Infiltration Exposed Through Data” racked up millions of views and algorithmic promotion.
In each case, outrage wasn’t a byproduct – it was the fuel.
And platforms didn’t just watch. They profited.
Algorithms are optimized to keep us engaged. That means prioritizing content that shocks, enrages, and divides. And hate? It performs spectacularly. It gets clicks, shares, comments. It triggers emotions that hook users – and advertisers. In the logic of the attention economy, hate isn’t a glitch. It’s the feature.
Social platforms don’t necessarily endorse hate. But they don’t need to. All they need is engagement. And hate delivers that in spades.
The Algorithm Doesn’t Care – But It Knows What You’ll Click
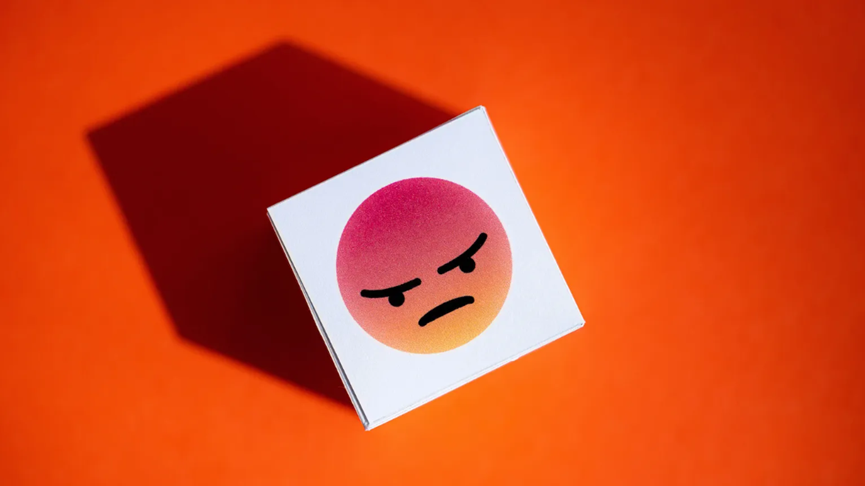
This phenomenon is not accidental, nor isolated. As Matamoros-Fernández (2017) articulates in her theory of platformed racism, digital platforms embed racialized hierarchies into their very logic of visibility. Hate speech is not just tolerated, it is algorithmically rewarded when it is controversial enough to spark interaction. The system promotes what polarizes because polarization produces data.
Similarly, Adrienne Massanari’s (2017) study of Reddit reveals how algorithmic sorting and community norms work together to produce what she calls a “toxic technoculture.” The design does not simply allow hateful content—it invites, organizes, and rewards it.
TikTok reflects the same structure: what provokes, spreads. What divides, thrives.
The algorithm isn’t showing you what’s true — it’s showing you what keeps you scrolling.
In another words, the algorithm isn’t neutral. It doesn’t judge fairly like a referee — it sells like a street vendor, yelling whatever gets your attention fastest.
Academic studies have peeled back the code. In one 2023 experiment by Milli et al., Twitter’s engagement-ranking algorithm showed users political posts where over 60% expressed anger and 46% included out-group hostility. When users switched to chronological order, those numbers dropped dramatically. The algorithm prefers outrage.
Another study by Huszár et al. found that Twitter’s feed algorithm amplifies specific political views disproportionately — not because they’re more “true,” but because they drive more interaction. You’re not really choosing what to see. The algorithm is choosing what it thinks you’ll react to.
Why? Because emotional content keeps you scrolling. A post that triggers your moral outrage will keep you on the app longer. The angrier you are, the more you click. The more you click, the more ads you see. The more ads you see, the more money they make.
The algorithm doesn’t care about truth. But it knows who you like to hate. And that’s enough.
Outrage Pays: Why Hate Is an Asset, Not a Bug
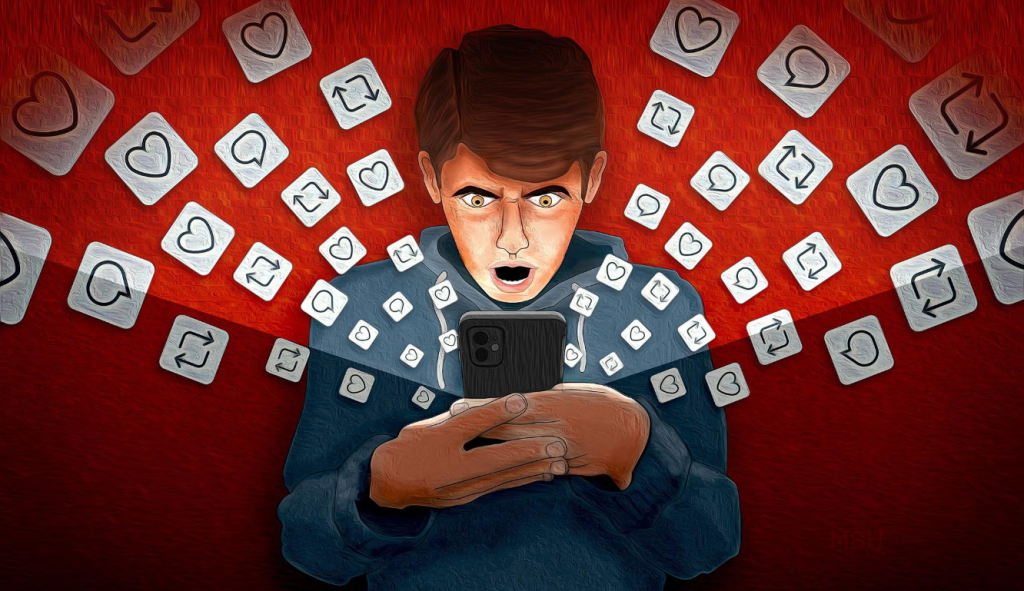
Behind the platform’s dashboard, one god rules all: Engagement. Likes, shares, comments, time-on-site — these are the algorithm’s currency. The more a piece of content provokes action, the more it gets pushed to others.
This is what’s known as attention monetization — platforms convert user attention into advertising revenue by maximizing time spent and interaction rates (Wu, 2016).
Terry Flew (2021) notes that platforms face a conflict between public responsibility and profit: they host speech, but also monetize its amplification. Hate goes viral not in spite of the system, but because of it.
Sinpeng et al. (2021) revealed that Facebook in Asia-Pacific often applied reactive moderation, especially in linguistically complex or geopolitically sensitive regions. Enforcement was patchy, but hate continued to earn engagement.
When anger equals engagement, and engagement equals profit — hate becomes a business model, not a mistake.
The result? Hate isn’t suppressed — it’s rewarded.
Facebook whistleblower Frances Haugen revealed that the company’s internal studies found ways to reduce hate speech by tweaking the algorithm. But the cost was lower engagement. “If we made the platform kinder,” she testified, “people would click less.” So the company chose to leave the anger dial turned up — even if it meant more extremism, more division, and more harm.
This isn’t a glitch.
It’s the business model.
Too Late to Moderate – The Machine Has Already Decided
Every time a platform is accused of enabling hate speech, the corporate response is familiar:
“We’ve taken the content down.”
But that’s like saying “We brought the fire extinguisher — after the house burned down.”
Sarah Roberts (2019) frames content moderation as “invisible labor,” always a step behind the viral machine. Hate content, once spread, is rarely undone by deletion.
Meta’s policies may appear balanced, but often ambiguity in enforcement enables borderline hate speech to flourish. “Just joking” or “ironic” framing allows plausible deniability—until harm is already done.
Meta’s transparency reports show that “newsworthiness” or “discussion value” can delay intervention.
In effect, this means hate gets a window to go viral before moderation reacts.
Because hate speech doesn’t start with a “violation report.” It starts with a recommendation.
According to Amnesty (2022), Facebook’s algorithm actively promoted Burmese-language hate content to thousands of users — before anyone even flagged it. The harm was already done before the moderators showed up.
Moderation is always chasing behind. No matter how fast you delete a post, you can’t outrun the speed of algorithmic distribution. It’s like trying to shut a dam after the flood’s already destroyed the village.
In Sri Lanka’s case, the false rumor about Muslims “poisoning food” spread to tens of thousands within hours. Facebook only responded two days later — by then, shops had been burned and people were injured. As one former employee put it:
“The algorithm moves faster than any human. And it doesn’t care about right or wrong.”
So yes, platforms are improving their moderation. But the real decisions are made not by human moderators, but by a machine — and that machine has already decided to show you the most enraging content it can find.
Case Study – TikTok, Trends, and the Viral Spiral of Hate
Stylized Outrage: How TikTok Turns Hate into Entertainment
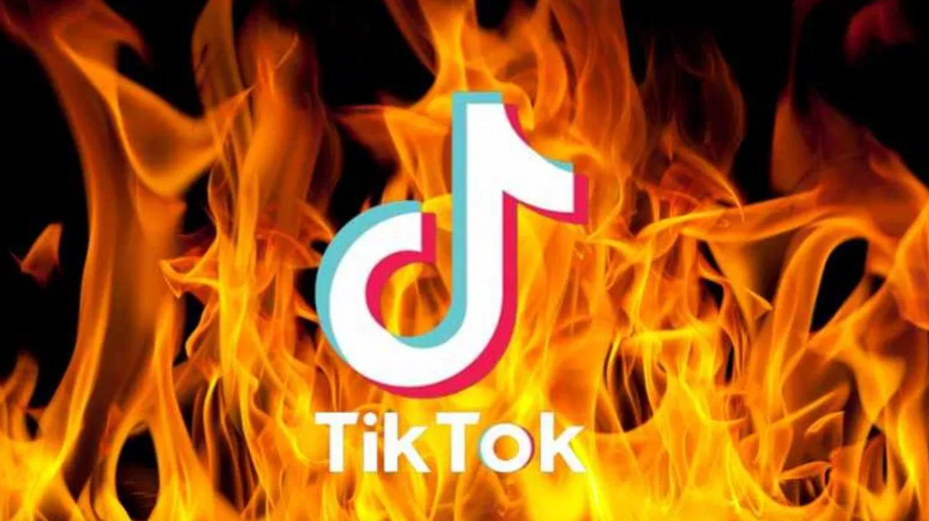
We’ve talked a lot about Facebook’s involvement in the Rohingya crisis, YouTube’s rabbit holes of extremism, and Twitter’s role in political polarization. But if you think we’re done unpacking the worst of platform design, think again.
Because now, it’s TikTok’s turn — and it might just be the most dangerous yet.
TikTok isn’t just the world’s most downloaded app. It’s where Gen Z lives, creates, and consumes culture. It’s also where hate can go viral — not despite the platform’s design, but because of it.
Let’s rewind to early 2023. A wave of videos with the hashtag #WhiteLivesMatter started gaining serious traction. Some were blatant white supremacist rants. Others were… slicker. They hid racist tropes behind humor, emoji-laced captions, dramatic music, or ironic filters. One video even used vintage historical footage to paint a not-so-subtle picture: that white culture was “under attack.”
And no — people weren’t searching for this stuff.
It was showing up for them.
That’s TikTok’s algorithm at work. The For You Page doesn’t care what you believe — only how long you watch. If you linger, rewatch, swipe back, or leave a comment (even a critical one), the system reads it as “interest.” Then it gives you more of the same.
This is what the ISD calls a feedback loop: emotional triggers → engagement → algorithmic reward → more emotional triggers.
The more you react, the more it shows up.
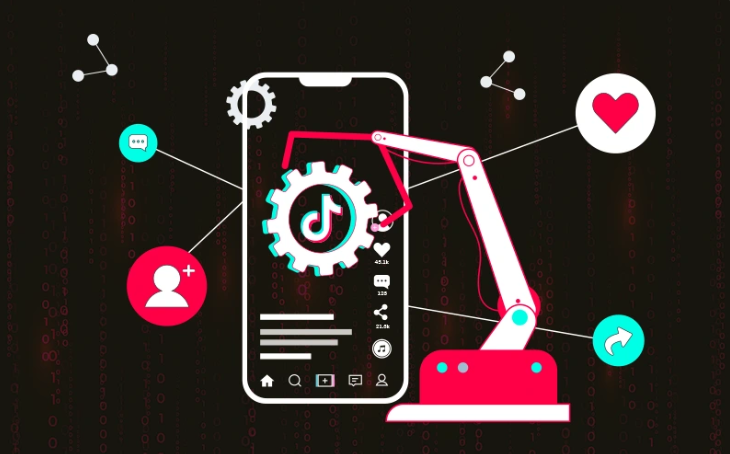
Sound familiar? That’s the attention economy in motion. TikTok’s entire ecosystem is built to keep you swiping, keep you watching. And what does that better than outrage, anxiety, or hate?
As Wu (2016) put it: platforms don’t sell ads — they sell attention. And emotion is what grabs it.
But here’s what makes it worse: TikTok packages hate in aesthetics. It’s got filters. Soundtracks. Humor. Irony. Racism doesn’t look like a rant anymore — it looks like a meme.
A 2023 study by the Center for Countering Digital Hate (CCDH) revealed that TikTok’s algorithm showed hate-related content to new users within 30 minutes of account creation — especially if the user engaged with nationalist, anti-immigrant, or misogynistic keywords. More alarmingly, hate hashtags were often accompanied by aestheticized video formats (filters, music, memes), making harmful content emotionally persuasive, even entertaining.
TikTok responded by banning some hashtags and suspending accounts. But the design problem remains: the system rewards what triggers — not what informs. Unlike fact-based content, outrage travels fast and embeds deeper because it aligns with emotional patterns of scrolling behavior.
“We are seeing a new kind of propaganda: stylized, optimized, and gamified for the scroll,” said CCDH researcher Callum Hood. “And TikTok’s algorithm is the perfect distributor.”
Callum Hood from the Center for Countering Digital Hate (CCDH) called this “viral hatewear”:
“On TikTok, hate isn’t hidden. It’s glorified.”
TikTok did remove some of the original videos after backlash. But thousands of variations stayed online — dodging detection with misspelled hashtags, remixes, or private shares.
Roberts (2019) calls this the “whack-a-mole” problem: no amount of manual moderation can beat an algorithm that’s already done its job.
And legally? We’re not quite catching up.
The EU’s Digital Services Act now requires platforms like TikTok to audit their recommender systems.
Australia’s Online Safety Act 2021 gives regulators the power to demand algorithm transparency.
But TikTok’s responses have been partial at best.
And the U.S.? Still no federal laws targeting recommendation algorithms.
So here’s the takeaway: TikTok isn’t just letting hate exist.
It’s letting hate perform.
Wrapped in trends, sugar-coated in memes, and delivered by the most efficient attention engine we’ve ever built.
If Facebook let hate go viral, and YouTube radicalized your uncle, then TikTok is teaching your cousin how to dress up racism for likes.
Don’t Just Moderate the Fire — Rethink What Fuels It
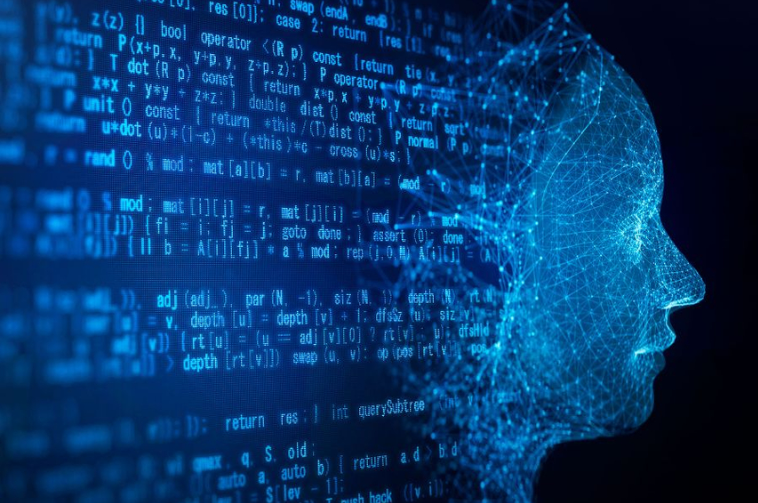
TikTok’s case makes one thing clear: removing content isn’t enough. Unless platforms change the rules that make hate viral, moderation will always be too little, too late.
We need structural reform — starting with how algorithms rank content. Scholars like Tufekci (2022) and Gillespie (2020) argue for algorithmic pluralism — designing systems that prioritize quality and public value, not just clicks. That means slowing down the spread of unverified content and boosting balanced voices.
Platforms should also give users more control — like toggling between algorithmic and chronological feeds — and explain why a post appears at all. Right now, the feed often feels like manipulation, not personalization.
And let’s not forget the business model. So long as profit depends on outrage, hate will always have a home. Some platforms are testing alternatives, like community governance or subscription models — early steps, but steps nonetheless.
Governments are catching on too. The EU’s Digital Services Act now mandates recommender system audits, and Australia’s Online Safety Act pushes for algorithmic transparency. But real accountability takes more than paperwork.
Hate doesn’t disappear when you delete a post — not if the system keeps rewarding it.
In an age where platforms profit from outrage and algorithms thrive on division, deleting hateful posts is not enough. Hate doesn’t go viral by accident — it goes viral because the system is designed to reward it. Unless we change how the system defines “value,” any moderation will remain cosmetic, not structural.
To move forward, platforms must prioritise algorithmic reform, transparency, and responsibility over engagement-at-all-costs. As long as business models depend on emotional manipulation, hate will continue to sell. Real change means reshaping what’s amplified — and reimagining the feed before it feeds the fire.
References
Amnesty International. (2022). The social atrocity: Meta and the right to remedy for the Rohingya. Amnesty International. https://www.amnesty.org.uk
Beech, H. (2022, September 29). Facebook’s role in Myanmar genocide detailed in new report. Time. https://time.com/6217730/myanmar-meta-rohingya-facebook/
Big Think. (n.d.). Why outrage is so addictive — and what you can do about it. Big Think. https://bigthink.com/thinking/outrage/
Bloomberg. (2020, May 13). Facebook apologises for ‘misuse of platform’ that led to Sri Lanka’s deadly anti-Muslims riots in 2018. South China Morning Post. https://www.scmp.com/news/asia/south-asia/article/3084148/facebook-apologises-misuse-platform-led-sri-lankas-deadly-anti
Dhulipala, S. (2023, September 27). The echo chamber effect: How algorithms shape our worldview. Campaign Asia. https://www.campaignasia.com/article/the-echo-chamber-effect-how-algorithms-shape-our-worldview/491762
DW News. (2021, August 24). How Facebook failed the Rohingya | DW News [Video]. YouTube. https://www.youtube.com/watch?v=lVaHR9KCicA
Flew, T. (2021). Hate speech and online abuse. In Regulating platforms (pp. 91–96). Cambridge: Polity Press.
Grey, N. (2025, February 7). How does TikTok work: TikTok algorithm explained. Social Followers UK. https://www.socialfollowers.uk/blogs/how-the-tiktok-algorithm-works-guide-for-uk/
Huszár, F., Ktena, S. I., O’Brien, C., Belli, L., Schlaikjer, A., & Hardt, M. (2022). Algorithmic amplification of politics on Twitter. Proceedings of the National Academy of Sciences (PNAS), 119(25), e2023301118. https://doi.org/10.1073/pnas.2023301118
Matamoros-Fernández, A. (2017). Platformed racism: The mediation and circulation of an Australian race-based controversy on Twitter, Facebook and YouTube. Information, Communication & Society, 20(6), 930–946. https://doi.org/10.1080/1369118X.2017.1293130
Massanari, A. (2017). #Gamergate and The Fappening: How Reddit’s algorithm, governance, and culture support toxic technocultures. New Media & Society, 19(3), 329–346. https://doi.org/10.1177/1461444815608807
Milli, S., Carroll, M., Wang, Y., Pandey, S., Zhao, S., & Dragan, A. (2023). Engagement, user satisfaction, and the amplification of divisive content on social media. PNAS Nexus, 2(3), pgad062. https://doi.org/10.1093/pnasnexus/pgad062
Roberts, S. T. (2019). Behind the screen: Content moderation in the shadows of social media. New Haven: Yale University Press.
SBG San Antonio. (2023, January 4). 12-year-old boy battles life-threatening burns after TikTok fire challenge goes horribly wrong. CBS Austin. https://cbsaustin.com/news/nation-world/12-year-old-boy-battles-life-threatening-burns-after-tiktok-fire-challenge-goes-horribly-wrong-tuscon-arizona-hospital-ambulance-therapy-skin-grafts-surgeries
Sinpeng, A., Martin, F., Gelber, K., & Shields, K. (2021). Facebook: Regulating hate speech in the Asia Pacific. University of Sydney & University of Queensland. https://r2pasiapacific.org/files/7099/2021_Facebook_hate_speech_Asia_report.pdf
Törnberg, P. (2022). How digital media drive affective polarization through partisan sorting. PNAS, 119(50), e2207159119. https://doi.org/10.1073/pnas.2207159119
UK Parliament. (2023). Online Safety Bill. https://bills.parliament.uk/bills/3137
Vaidhyanathan, S. (2018). Antisocial media: How Facebook disconnects us and undermines democracy. New York: Oxford University Press.
Wu, T. (2016). The attention merchants: The epic scramble to get inside our heads. New York: Knopf.
YaleNews. (2021, August 13). ‘Likes’ and ‘shares’ teach people to express more outrage online. Yale University. https://news.yale.edu/2021/08/13/likes-and-shares-teach-people-express-more-outrage-online
Be the first to comment