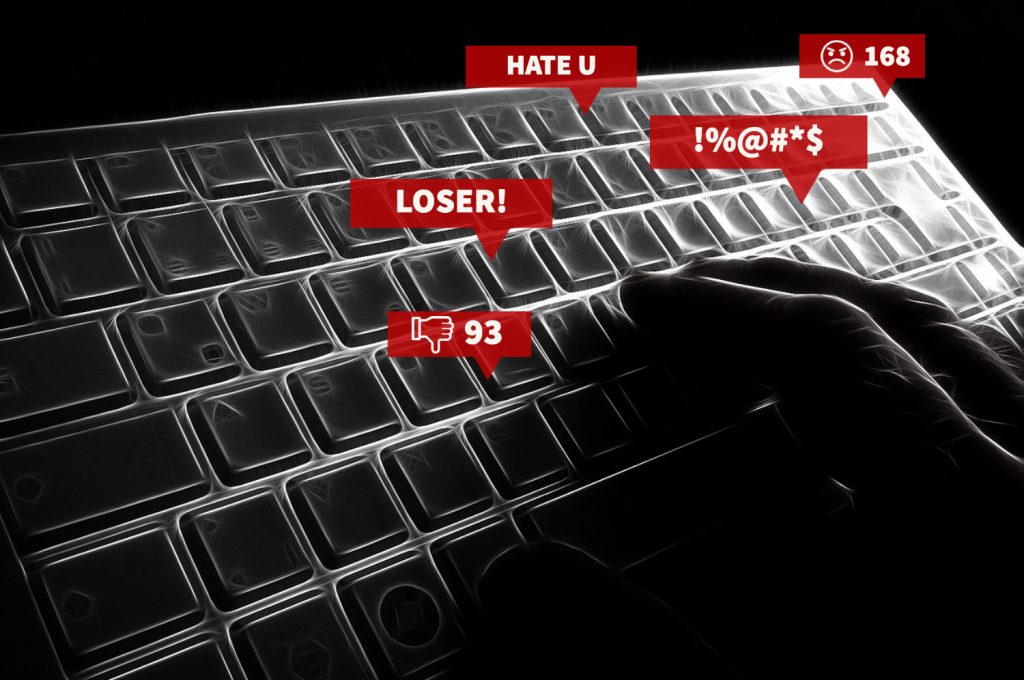
In the digital age, social platforms have become the main place for people to exchange ideas, share information and express themselves. However, open platforms also bring some side effects, one of which is the spread of online hate speech. Online hate speech is any speech that is intended to disparage, exclude or incite violence and hatred against a specific person or group based on race, religion, gender, sexual orientation or other characteristics (Citron & Norton, 2011). Such remarks not only cause psychological harm to the person being attacked, but may also trigger offline violence and pose a threat to social stability (Flew, 2021).
The impact and challenges of online hate speech
Digital platforms play a complex and critical role in managing hate speech. On the one hand, they are highly expected to be able to effectively identify and limit the spread of hate speech and protect users from harm; on the other hand, they also face the challenge of finding a balance between combating hate speech and protecting freedom of speech (Citron & Norton, 2011). Furthermore, as technology evolves and Internet usage increases worldwide, these platforms must operate within different cultural, legal, and social contexts, making it more difficult to develop unified and effective hate speech management strategies (Tufekci, 2017). Despite these challenges, digital platforms have a responsibility and an opportunity to create safer and more inclusive online environments. How they respond to this responsibility not only affects the development of the platform itself, but also has a profound impact on the health and progress of the entire society. In the process of managing hate speech online, digital platforms face multiple challenges that not only involve technical and legal issues, but also go to the core of moral and social values (Gillespie, 2018). Digital platforms must find a delicate balance between combating hate speech and protecting free speech. As Flew (2021) pointed out, overly strict content management policies may restrict free speech and inhibit important social dialogue; allowing hate speech to spread will harm users and destroy social harmony. The search for this balance is complex and challenging, especially in the context of globalization, where different cultures and societies have vastly different views on what constitutes hate speech (Wenguang, 2018).
The balance between AI review and manual review
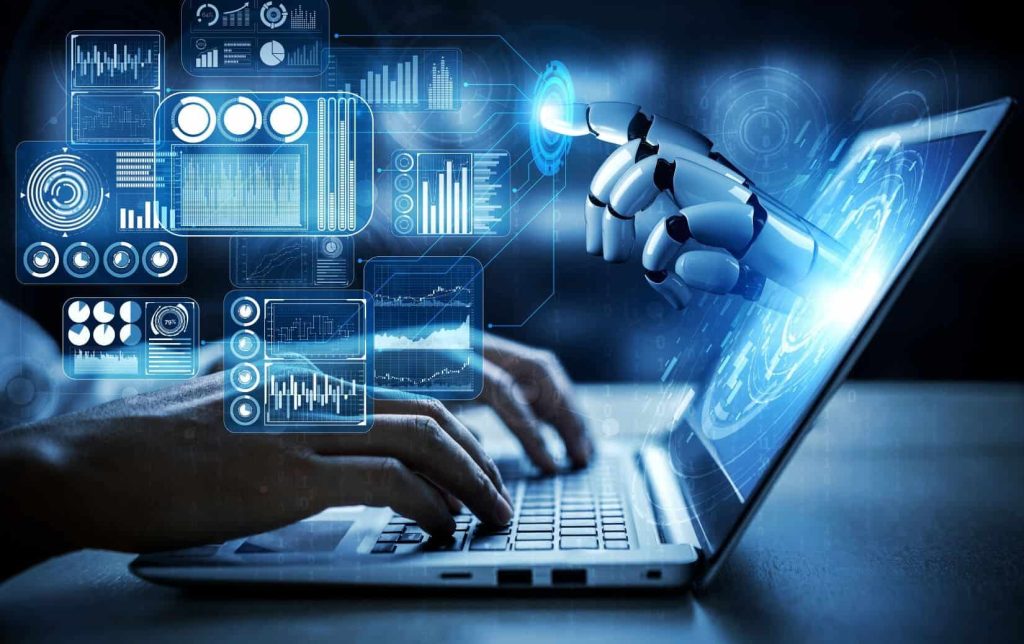
During the 2020 U.S. presidential election, Twitter made changes to its content moderation policies to reduce the spread of misinformation and hate speech (Roberts, 2019). Among them, measures such as labeling warnings and restricting forwarding of tweets by specific political figures have triggered widespread discussions. While these actions were intended to reduce the spread of hate speech and false information, they also sparked debate about free speech. Critics argue that as a social media platform, Twitter should remain neutral and should not interfere with political speech, even if that speech may be misleading or radical (Roberts, 2019). The case highlights the delicate balance digital platforms have to navigate between keeping public spaces of dialogue clean and protecting free speech. On the one hand, the unrestricted spread of misleading information and hate speech may cause harm to the democratic process and social harmony; on the other hand, overly strict content management measures may be regarded as an infringement of freedom of expression (Rao, 2020). These platform actions and policy changes inspire a rethinking of the boundaries of free speech in the digital age.
Artificial intelligence is being used as a new tool in content moderation to identify misinformation as well as hate speech. While artificial intelligence and machine learning technologies have made progress in automatically identifying hate speech, technical limitations remain a significant challenge. As discussed by Citron and Norton (2011), algorithms may have difficulty accurately identifying subtle differences in context, such as sarcasm, humor, or culturally specific expressions. This technical inadequacy can lead to misjudgments, either missing genuine hate speech or incorrectly restricting harmless speech. YouTube uses complex algorithms to automatically identify and address content that violates its community guidelines, including hate speech. However, technical limitations lead to problems with false determinations. For example, there are reports that some history education channels have been wrongly labeled as hateful for showing World War II-related content (Suzor, 2019). This case illustrates the limitations of algorithms in automatically identifying hate speech, especially when complex contextual and cultural contexts need to be understood. Wrong determination will not only lead to unfair treatment of content creators, but may also lead to inappropriate restrictions on educational information content, thus damaging the information diversity and educational value of the platform. Therefore, digital platforms must not only continuously improve technical algorithms, but also find an effective balance between automation and manual review.
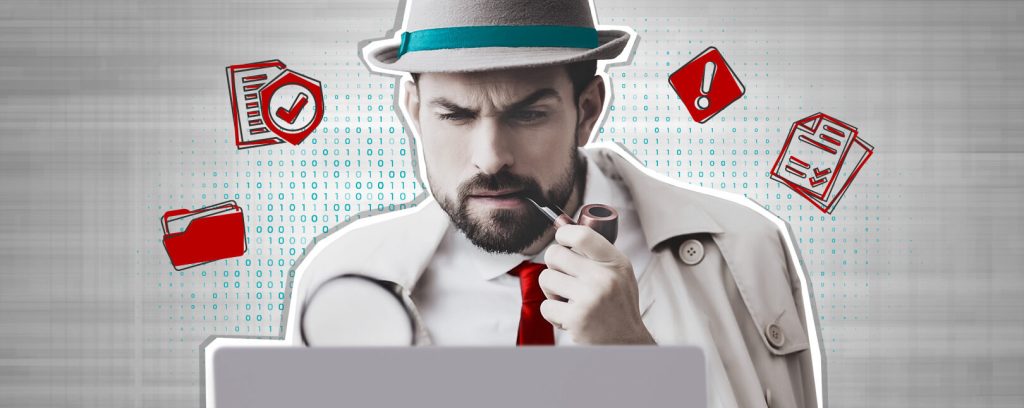
Although manual review does not have the shortcomings of possible misjudgments identified by artificial intelligence, the feasibility of this method and the psychological impact on reviewers are also issues worthy of attention. Content moderators who are exposed to hate speech for extended periods of time may experience severe psychological stress and trauma. In addition, with the massive growth of user-generated content, a model that relies on manual review of all content is not only expensive but also difficult to implement. As the world’s largest social networking platform, Facebook relies on thousands of content moderators to check and deal with illegal content, including hate speech (Sinpeng et al., 2021). However, the working conditions and mental health of these auditors have raised public concerns. In 2019, a series of reports revealed the extreme work pressure faced by Facebook content moderators, including prolonged exposure to violent, abusive and hateful content, leading many to develop mental health issues such as post-traumatic stress disorder (Roberts, 2019). Additionally, Facebook has faced lawsuits and public pressure to provide adequate mental health support and improvements to its work environment. This illustrates the challenges of relying on manual moderation of online content, particularly the mental health impact on staff dealing with hate speech and other harmful content. While manual moderation is one of the effective means of identifying and dealing with hate speech, the nature of this work requires platforms to provide adequate mental health support, create a healthy work environment, and take measures to reduce the psychological burden on moderators. Platforms need to pursue content management efficiency while also being responsible for the health and well-being of their employees. In addition, this situation also reflects the urgent need to improve AI content review technology to make AI more humane and efficient in managing content, so as to reduce reliance on manual review, alleviate the psychological pressure faced by manual review, and ensure the safety and health of the community.
Opportunities for AI moderationtechnology

In the challenge of managing online hate speech, digital platforms not only have to face various difficulties. Due to the many problems currently existing in AI moderation, trying to balance the relationship between manual and AI moderation is also a major challenge faced by digital platforms. However, technological innovation, educational cooperation, etc. have also brought opportunities and inspiration for digital platforms to contribute in this regard. Jigsaw is a technology incubator owned by Google that aims to solve the world’s most pressing security issues, including online hate speech. Jigsaw has developed a range of tools, including “Perspective,” a tool based on machine learning technology that can identify and filter hate speech and harmful comments (Jigsaw, n.d.). By training its language and cultural expertise, Jigsaw continues to refine its algorithms to more accurately identify hate speech in different cultural and linguistic contexts. This technology not only improves the accuracy of hate speech detection, but also reduces reliance on human moderation and their risk of exposure to harmful content.Facebook is also using advanced deep learning technology to improve the content moderation process on its platform. Unlike Jigsaw, this technology uses complex neural network models to identify and classify content containing hate speech and disinformation. Facebook’s AI system is trained with a large amount of diverse data and can more accurately understand and predict the potential risks of content in different contexts and cultural backgrounds (Gillespie, 2020). This technological advancement not only improves the accuracy of hate speech detection, but also increases the speed of processing big data, allowing platforms to quickly intervene at the beginning of information dissemination to prevent the spread of harmful content. Although it still faces problems of data bias and misjudgment, Facebook continues to optimize its algorithm and strives to minimize unnecessary interference with free speech while protecting user experience (Gillespie, 2020). In addition, Facebook allows users to customize banned words, and content containing restricted words will be blocked. This method reduces the pressure of review to a certain extent, and these words can also further assist the improvement of the algorithm. However, technical solutions are not panacea, and their efficiency and accuracy are often limited by algorithm design and training data quality. Nonetheless, the attempts of Jigsaw and Facebook provide the possibility of automated and efficient management of hate speech in cyberspace. The further improvement of Jigsaw in the future will help enhance the ability of the entire Internet ecosystem to manage hate speech.
On the other hand, enhancing cross-platform collaboration is also a major opportunity for the further development of AI review technology. Globally, major social media platforms such as Twitter, YouTube and Facebook are joining forces to develop standardized AI tools to identify and manage online hate speech. By sharing data, research and technology solutions, these platforms create a collaborative ecosystem designed to improve the efficiency and accuracy of content moderation in their respective and online environments. For example, the Global Internet Forum to Counter Terrorism (GIFCT) is an organization composed of several large technology companies that aims to combat online terrorism and extremist content by sharing technical resources and strategies. This cross-platform cooperation not only helps standardize the definition and response measures of hate speech, but also accelerates the learning and progress of AI on a global scale to better respond to rapidly changing cybersecurity challenges (Conway et al., 2019).
Summary
In the digital age, social platforms face both challenges and opportunities in the task of managing hate speech online. While AI has demonstrated strong potential in automatically moderating content, it still has limitations in identifying and filtering hate speech, especially when dealing with cultural and contextual nuances. However, technological innovations such as Google’s Project Jigsaw and Facebook’s deep learning technology are continuing to advance, improving the accuracy of hate speech detection and reducing reliance on human review. These technological advances not only improve the efficiency of content management on the platform, but also provide faster intervention methods to prevent the spread of harmful content. In addition, through cross-platform cooperation, such as the Global Internet Forum on Counter-Terrorism (GIFCT), major social media platforms are jointly developing and applying standardized AI tools to manage and suppress online hate speech in a more standardized and efficient way. This cooperation accelerates global learning and progress of AI technology, demonstrating the huge potential and positive impact of AI technology in global cybersecurity challenges.
Reference
Citron, D. K., & Norton, H. (2011). Intermediaries and hate speech: Fostering digital citizenship for our information age. Boston University Law Review, 91, 1435-1468.
Conway, M., Khawaja, M., Lakhani, S., Reffin, J., Robertson, A., & Weir, D. (2019). Disrupting Daesh: Measuring Takedown of Online Terrorist Material and Its Impacts. Studies in Conflict & Terrorism. https://doi.org/10.1080/1057610X.2019.1596658
Flew, Terry (2021) Hate Speech and Online Abuse. In Regulating Platforms. Cambridge: Polity, pp. 91-96 (pp. 115-118 in some digital versions)
Gillespie, T. (2018). Custodians of the Internet : Platforms, Content Moderation, and the Hidden Decisions That Shape Social Media. Yale University Press,. https://doi.org/10.12987/9780300235029
Gillespie, T. (2020). Content moderation, AI, and the question of scale. Big Data & Society. https://doi.org/10.1177/2053951720933233
Jigsaw. (n.d.). Censorship. Retrieved from https://jigsaw.google.com/issues/#censorship
Rao, M. F. (2020). Hate Speech and Media Information Literacy in the Digital Age: A Case Study of 2018 Elections in Pakistan. Global Media Journal, 18(34), 1–10.
Roberts, S. T. (2019). Behind the screen : content moderation in the shadows of social media. Yale University Press. https://doi.org/10.12987/9780300245318
Sinpeng, A., Martin, F., Gelber, K., & Shields, K. (2021, July 5). Facebook: Regulating hate speech in the Asia Pacific. Final Report to Facebook under the auspices of its Content Policy Research on Social Media Platforms Award. Dept of Media and Communication, University of Sydney and School of Political Science and International Studies, University of Queensland. https://r2pasiapacific.org/files/7099/2021_Facebook_hate_speech_Asia_report.pdfLinks to an external site.
Suzor, N. P. (2019). Lawless: The Secret Rules That Govern Our Digital Lives (1st ed.). Cambridge University Press. https://doi.org/10.1017/9781108666428
Tufekci, Z. (2017). Twitter and tear gas : the power and fragility of networked protest. Yale University Press. https://doi.org/10.12987/9780300228175
Wenguang, Y. (2018). Internet Intermediaries’ Liability for Online Illegal Hate Speech. Frontiers of Law in China, 13(3), 342–356. https://doi.org/10.3868/s050-007-018-0026-5
Be the first to comment