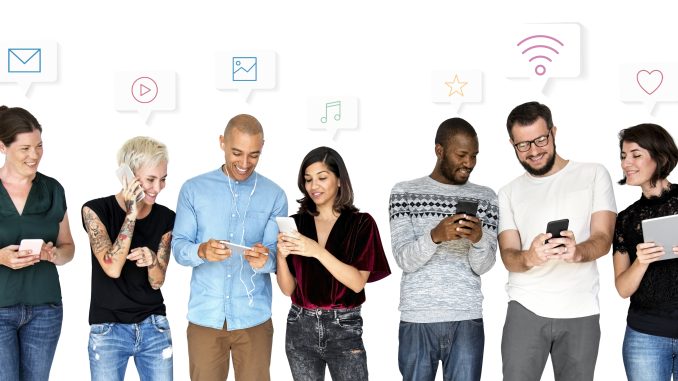
In the current era where technology intersects with everyday life, the implications of digital practices on privacy, equity, and access to services cannot be overstated. Profiling and digital redlining are two aspects of a larger digital ecosystem that perpetuates inequalities and poses significant challenges to digital policy and governance. The present blog post explores the concepts of profiling and digital redlining, delving into the case study of the threat posed by data-driven policing. It aims to uncover the implications of these practices for digital policy and governance. Overall, it is argued that digital profiling and redlining in data governance not only perpetuate historical inequalities but also challenge the very foundations of equity and justice in the digital age, necessitating comprehensive reforms in policy and governance to rectify these entrenched biases.
Overview of the Concepts
Profiling refers to the collection and analysis of data about individuals or groups to categorize them for various purposes, often leading to targeted actions or decisions (Allen, 2019). In the contemporary digital landscape, profiling can influence what advertisements, services, or information individuals are exposed to online.
Digital Redlining, drawing parallels from the historical practice of redlining in housing, involves discriminatory practices where services or information are denied or limited based on geographic location or demographic characteristics, often influenced by digital technologies (Allen, 2019).
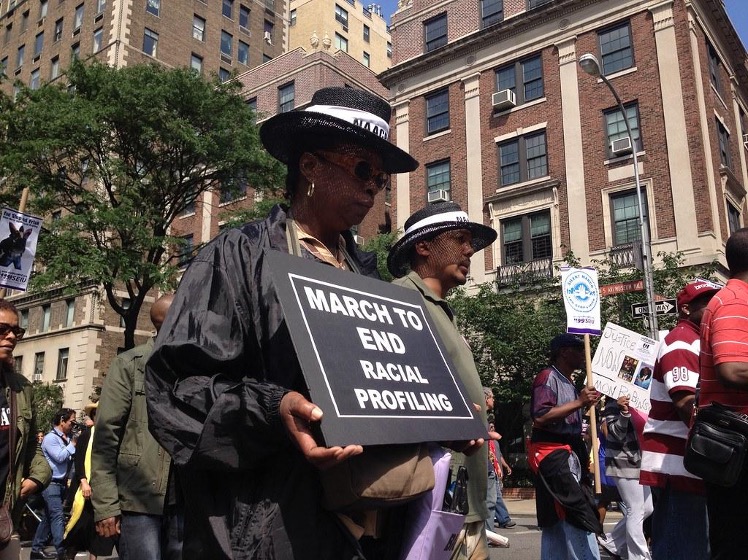
Synthesizing Insights from A Comparative Analysis: Historical Echoes in Modern Practices
Following the definitions, this segment will present the current scholarly findings that bridge these two pivotal concepts, offering a comparative and contrastive exploration to shed light on their implications in the digital era.
Firstly, Hill (2021) offers a broader historical lens, tracing the lineage of spatial racism from redlining to present-day practices. This research highlights how the legacies of spatial racism are not confined to the past but are actively recreated and reinforced through contemporary data-driven practices, illustrating a continuum of discriminatory practices that transcend time and technological advancements (Hill, 2021).
Lambright’s (2019) study of the Nextdoor app shows how digital platforms can continue exclusionary practices like those seen in suburban redlining from the mid-1900s. This means that these platforms can create spaces where racial profiling and segregation are not just accidental but important parts of how people interact. By copying these exclusionary practices digitally, it highlights how modern technologies can revive old biases, making “digital neighborhoods” that reflect the segregated world we see in physical spaces. (Lambright, 2019).
In the financial sector, Friedline and Chen (2021) highlight how fintech innovations, while promoting the democratization of financial services, often perpetuate economic disparities. They argue that digital redlining in the fintech space exacerbates access issues for marginalized communities, suggesting a digital translation of traditional redlining practices where systemic biases are embedded in algorithms that dictate who gets access to financial services and who does not (Friedline & Chen, 2021).
Allen (2019) extends the conversation to housing, spotlighting the insidious role of algorithmic decision-making in perpetuating housing segregation. This “algorithmic redlining” claims to be neutral and objective but actually carries on the biases of its creators. It shows how digital tools can continue old discrimination patterns (Allen, 2019).
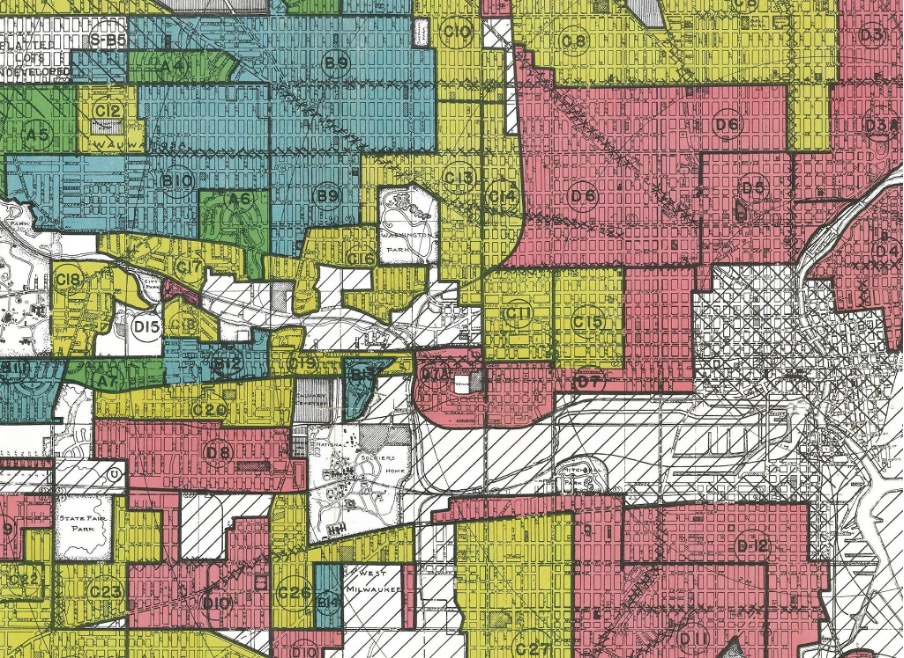
Image 2: A map of the city’s home purchases under redlining (WisContext, n.d.)
Across these studies, a common theme emerges: the digital and algorithmic reincarnations of redlining and profiling are not mere coincidences but are reflective of deeper systemic structures that have long leveraged spatial and informational boundaries to enforce and perpetuate inequality. Whether through the digital gating of community networks, the algorithmic biases in financial and housing sectors, or the historical continuities of spatial racism (Hill, 2021; Friedline & Chen, 2021; Allen, 2019), these practices illustrate how technology, far from being a neutral tool, is deeply enmeshed in the socio-economic fabric, often replicating and reinforcing long-standing disparities.
The comparison and contrast of these findings not only highlight the specificities of how digital and spatial redlining manifest across different sectors but also underscore a crucial insight: the reasons behind exclusion and discrimination, even though they show up in different ways, have the same origin in historical biases that still affect the world today.
Overview of the Selected Case: The Threat Posed by Data-Driven Policing
In Ángel Díaz’s (2021) case, “Data-driven policing’s threat to our constitutional rights,” the effects of predictive policing are examined, emphasizing how it adds to cycles of violence and keeps historical biases alive.
Díaz (2021) emphasizes that in the United Nations, the data-driven policing systems, claiming objectivity, unfairly target Black and brown communities by labeling them as high-crime areas in cities including Los Angeles, Chicago, and Phoenix. In particular, this is based on flawed data often derived from biased policing practices. The narrative delves into the negative consequences of relying on such data, noting instances where predictive policing has led to increased surveillance and unjust treatment of individuals, especially the youth in these communities. The discussion also considers the wider impact on constitutional rights, highlighting that data-driven policing undermines the principles of fair treatment and equal protection under the law. Díaz (2021) calls for a reevaluation and overhaul of these systems, advocating for a shift towards more equitable and just policing practices that do not compromise fundamental rights and freedoms.
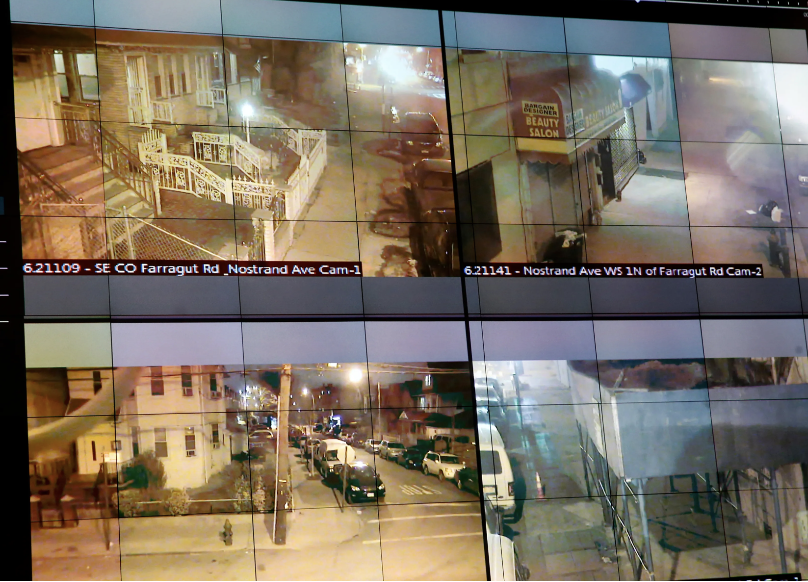
Image 3: Surveillance of neighborhoods and streets (Díaz, 2021)
Analysis of the Case and Articles
The case of predictive policing, as presented by Díaz (2021), demonstrates a poignant illustration of how digital tools, meant to advance law enforcement efficiency, can inadvertently perpetuate historical biases, particularly against marginalized communities. This digital incarnation of redlining, where certain neighborhoods are disproportionately targeted based on historical crime data, underscores a modern dilemma: the intersection of technology with entrenched social inequities.
Díaz (2021) brings to light the recursive nature of bias in predictive policing. That is, systems trained on historical data can inadvertently reinforce the very biases they might aim to eliminate, creating a feedback loop that cements the prejudicial status quo. Such a system does not just fail in its mission to be objective but also erodes the trust between law enforcement and the communities they serve, particularly affecting black and brown populations who find themselves disproportionately scrutinized.
Further implying this point are cases from various cities like Los Angeles, Chicago, and Phoenix, which Díaz (2021) references to demonstrate the tangible repercussions of predictive policing. This case study not only reveals the system’s failures in those cities but also highlights a broader issue: digital redlining extends beyond policing, affecting individuals’ access to essential services like education, housing, and employment. Such multifaceted impacts of digital redlining exemplify how deeply embedded these biases can become, influencing various aspects of life and perpetuating a cycle of discrimination.
While Díaz’s (2021) case is insightful, broader academic literature, as aforementioned, further contextualize this issue. These academic discoveries expand on the understanding of the ramifications of digital redlining across multiple industries, while also supporting Díaz’s (2021) findings. They prompt a reexamination of the part played by data and algorithms in maintaining societal prejudices and call on technologists, legislators, and community leaders to take a comprehensive approach to addressing these deeply ingrained problems. These viewpoints’ confluence provides a more complete picture of the digital redlining phenomena and emphasizes the urgent need for multifaceted approaches to remove these technological barriers and promote social justice.
Key Issues in Digital Policy and Governance
The case of predictive policing within the framework of digital redlining highlights several critical issues in digital policy and governance:
- Data Integrity and Bias: The reliance on historical crime data, often tainted by systemic biases, underscores the need for mechanisms to ensure data integrity and mitigate bias in algorithmic decision-making.
- Transparency and Accountability: There is a pressing need for greater transparency in how predictive policing algorithms are developed and deployed, alongside mechanisms for accountability to address any biases or errors in these systems.
- Community Impact and Engagement: Understanding and addressing the community-level impacts of predictive policing are crucial. This involves engaging with communities to gain insights into the real-world implications of these technologies and to foster trust and collaboration.
- Policy Frameworks and Regulations: It is crucial to create strong rules and regulations to make sure predictive policing technologies are used ethically. This ensures they benefit the public without violating individual rights or making existing injustices worse.
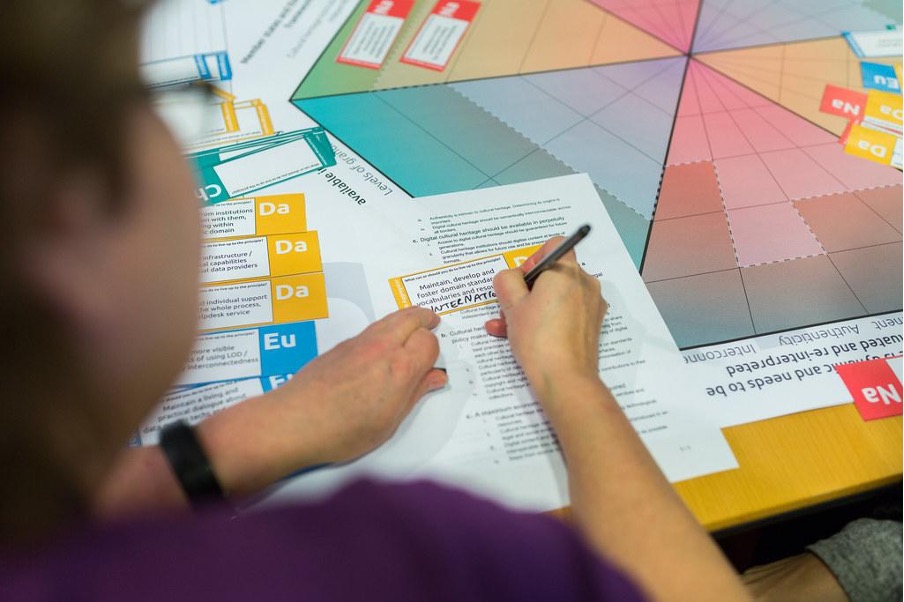
Image 4: Stock image from Freepik
Implications of the Concepts and Findings
For addressing the issues that are identified, the scholarly articles discussed reveal a multifaceted view of how digital technologies, while heralding advancements and efficiencies, simultaneously perpetuate long-standing biases and inequalities. These findings have significant implications for digital policy and governance, requiring a comprehensive and multifaceted response.
- Inclusive Policy-making: The analysis of the case underscores the need for policies that do not merely address the manifestations of digital redlining and profiling but tackle the root causes. For instance, Lambright (2019) demonstrates how digital platforms like the Nextdoor app can foster exclusionary communities, echoing mid-century suburban exclusivity, which underscores the necessity for policies that promote inclusivity and diversity in digital communities. Similarly, Friedline & Chen’s (2021) exploration of fintech emphasizes the need for financial regulations that prevent digital technologies from exacerbating economic disparities, ensuring that fintech serves as a tool for economic inclusion rather than exclusion.
- Transparency and Accountability: The call for transparency is particularly salient in the context of Allen’s (2019) discussion on algorithmic redlining in housing. By making algorithms transparent, stakeholders can identify and rectify biases inherent in automated decision-making processes. This aligns with the broader need for accountability in how digital technologies are deployed, as evidenced by Hill’s (2021) examination of data-driven neighborhood classification, which can perpetuate spatial racism if left unchecked.
- Community Engagement: Engaging communities, especially those marginalized, in the design and governance of digital technologies is crucial. This participatory approach can ensure that digital technologies reflect the diverse needs and concerns of all citizens. For example, by involving communities in shaping how neighborhood social networks operate, as discussed in Lambright’s (2019) analysis, platforms can be designed to foster genuine inclusivity and prevent the digital replication of historical biases.
- Educational Initiatives: In order to enable people and communities to identify and confront digital redlining and profiling, education and awareness-raising are crucial. Individuals can more effectively advocate for just and equitable digital practices when they are more aware of the potential biases in digital technologies, as the scholar perspectives revealed. To promote a shared commitment to addressing and redressing the injustices ingrained in digital systems, this education ought to be extended to legislators, engineers, and the general public.
By addressing these implications, policymakers, technologists, and communities can work together to ensure that the digital landscape is equitable and inclusive, preventing the perpetuation of historical biases and promoting a more just digital future.
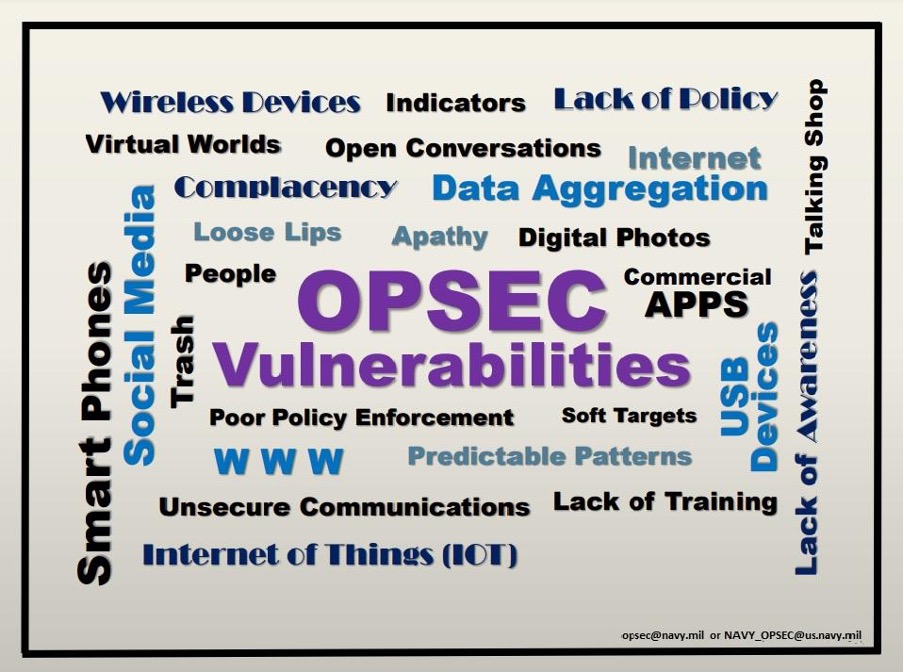
Image 5: NAVIFOR OPSEC Info graph (DVIDS, n.d.)
Conclusion
The combination of predictive policing and digital redlining provides a detailed example of the difficulties where technology, data, and social justice intersect. As society depends more on data-driven algorithms for decision-making in policy and governance, it is crucial to carefully assess these tools. This is to avoid continuing historical biases and to promote fairness and justice. Both the case study and academic discussions highlight the importance of tackling these issues promptly. They call for a balanced approach that maximizes technology’s benefits while guarding against its potential negative impacts.
In other words, as we navigate the digital age, the intersection of technology with societal biases presents challenges, especially in the case of the data-driven policing systems, that require a concerted effort from policymakers, technologists, and the community. Understanding and addressing the issues of profiling and digital redlining are crucial steps toward a more equitable and inclusive digital future.
I invite you to share your thoughts and experiences related to digital profiling and redlining. How do you think we can collectively address these challenges to create a more inclusive digital landscape? Your insights are valuable in shaping the discourse around digital equity and governance.
References
Allen, J. A. (2019). The Color of Algorithms: An Analysis and Proposed Research Agenda for Deterring Algorithmic Redlining. The Fordham Urban Law Journal, 46(2), 219-270. https://go.gale.com/ps/i.do p=AONE&u=usyd&id=GALE%7CA585353630&v=2.1&it=r&aty=sso%3A+shibboleth
Díaz, Á. (2021, September 13). Data-driven policing’s threat to our constitutional rights. Brookings. https://www.brookings.edu/articles/data-driven-policings-threat-to-our-constitutional-rights/
Friedline, T., & Chen, Z. (2021). Digital redlining and the fintech marketplace: Evidence from US zip codes. The Journal of Consumer Affairs, 55(2), 366–388. https://doi.org/10.1111/joca.12297
Hill, A. B. (2021). Before Redlining and Beyond: How Data-Driven Neighborhood Classification Masks Spatial Racism. New York: Metropolitics. https://metropolitics.org/IMG/pdf/met-hill.pdf
Lambright, K. (2019). Digital Redlining: The Nextdoor App and the Neighborhood of Make-Believe. Cultural Critique, 103(103), 84-. https://doi.org/10.5749/culturalcritique.103.2019.0084
Images
Díaz, Á. (2021, September 13). Data-driven policing’s threat to our constitutional rights. Brookings. https://www.brookings.edu/articles/data-driven-policings-threat-to-our-constitutional-rights/
DVIDS. (n.d.). Assessment Navifor OPSEC Info Graph [Image]. DVIDS. Retrieved from https://www.dvidshub.net/image/6431265/assessment-navifor-opsec-info-graph
Freepik. Retrieved from https://www.freepik.com/free-photo/diverse-friends-using-their-devices_13301062.htm#fromView=search&page=2&position=30&uuid=140db5cc-ad8d-4453-92ac-0efff772e14a
Stop and Frisk Appeal Déjà Vu. (2014, October 23). DrexelNow. https://newsblog.drexel.edu/2014/10/23/stop-and-frisk-appeal-deja-vu/
WisContext. (n.d.). How redlining continues to shape racial segregation in Milwaukee. WisContext. Retrieved from https://wiscontext.org/how-redlining-continues-shape-racial-segregation-milwaukee
Be the first to comment