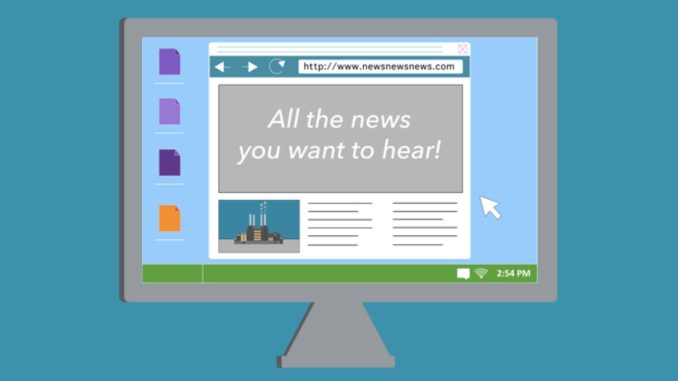
Navigating the Digital Maze – Datafication, Algorithms, and Personalized Content
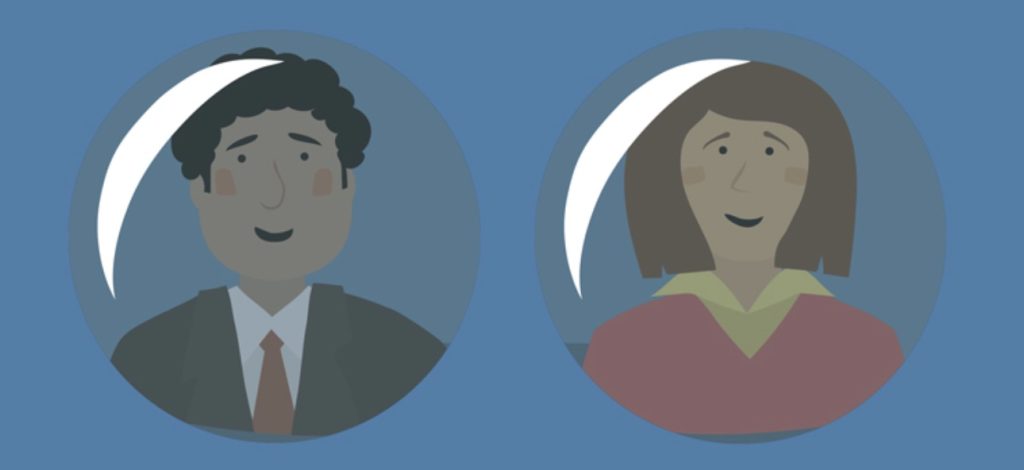
Introduction
Recently, a scene like this appeared on Chinese social media “red book”. A user posted their experience of beautifying black on the platform, attached photos of themselves before beautifying black, and shared their insights. It is worth discussing that this was originally a post about sharing experiences, but the following comments sparked intense discussions due to different aesthetic subjectivity. Some people who think that the blogger’s original skin color was beautiful generally believe that the skin after blackening does not match the mainstream Asian aesthetic, and they think that the blogger’s “blackening” behavior is imitating Western girls, and they find it difficult to understand the blogger’s behavior. The other people of the blogger’s beauty after getting black on the platform is positive, thinking that the blogger’s wheat colored skin is healthy and confident.In fact, people on Chinese media platforms find it difficult to coexist peacefully with different aesthetic concepts. There have always been two different voices opposing each other (healthy beauty and fair and thin beauty), and the “beauty” displayed on different social media platforms is also different. Nowadays, most media platforms adopt personalized recommendation algorithms and advocate that this algorithm can better bring users a better experience. I believe that depend on personalized recommendation algorithms, different voices and viewpoints are difficult to communicate with each other, resulting in the Echo Chamber effect and leading to the emergence of confirmation bias, exacerbating polarization between different groups. In fact, this algorithm has drawbacks that should not be ignored, and these drawbacks are affecting people’s lives. The information explosion in the Internet era has had a profound impact on our cultural experience (Andrejevic, 2019). I hope this article can remind people to approach the information they receive with a critical attitude in the context of digitalization.
Mechanisms for personalizing content
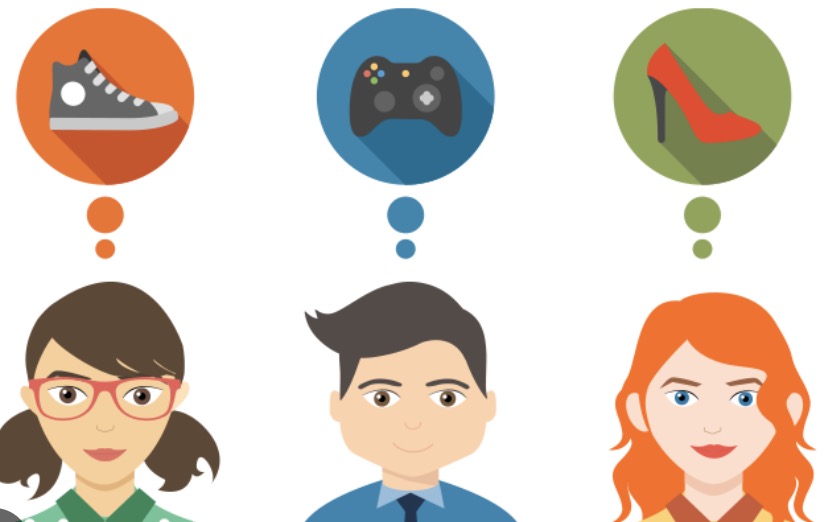
Social media platforms provide personalized recommendations to users based on their interests, preferences, and behaviors, using algorithms and data analysis to provide customized content. These contents can include news, articles, videos, advertisements, etc. that users are interested in (Andrejevic, 2019).
Social media platforms claim that personalized recommended content aims to:
1. Provide information that better aligns with user interests and provides a better user experience
2. Save users time and energy, help them quickly find the content they are interested in, avoid the search and filtering process in massive information, and save time and energy.
3. Promotion and sales effectiveness improvement. Social media platforms can recommend relevant advertisements and products to potential target users, improve the effectiveness of advertising and sales, and increase platform revenue.
4. Promote user participation and interaction, recommend relevant social circles, groups or users based on their interests and preferences, promote interaction and participation among users, and enhance the activity of social media platforms.
The role of big data: Big data delivers user data to personalized recommendation algorithms.
The most common data collection method for personalized recommendation mechanisms is to detect user online behavior and decode it for collection. This means that when users use Internet services, their behavior data will be recorded, such as browsing the web, clicking links, searching for keywords, purchasing goods, etc. These behavioral data can be collected and stored, and then processed and analyzed through personalized algorithms to provide a predictive basis for personalized recommendations.
Algorithm: Personalized engine
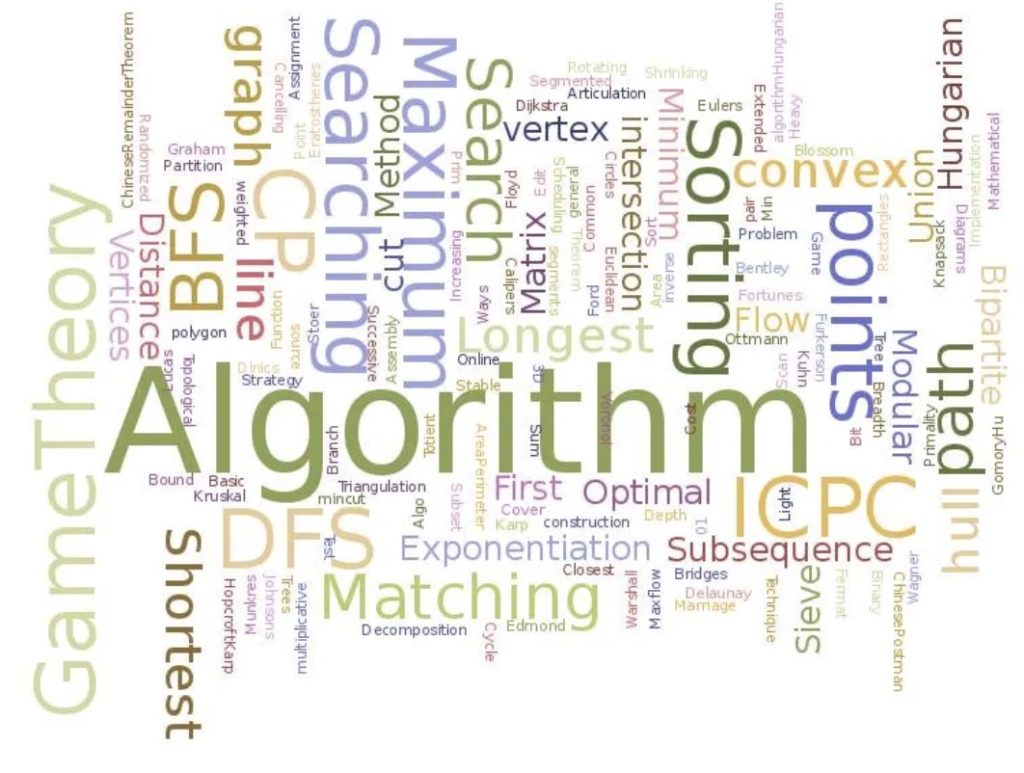
People need to pay attention to the use of algorithms in processing large datasets to provide personalized content. Firstly, the definition of personalized algorithms aims to provide users with personalized recommendations, services, or recommendations based on their personal characteristics and preferences, by analyzing and mining a large amount of user data. In other words, personalized algorithms are defined as automated, real-time, and context sensitive personalized selection processes that apply customized standards based on user characteristics, behavior, and location (Musto et al., 2012). They differ from traditional mass media selection mechanisms in that they are based on big data, including active consumer input and passive data, and allow users to act as second gatekeepers and data providers (Gilmanov, 2023).
So how do personalized algorithms handle large datasets?
Firstly, the algorithm will clean and preprocess the collected data, as large datasets often contain a large amount of noise and redundant information. Secondly, the algorithm will perform feature extraction and selection on user data, typically requiring useful features to be extracted from the original data. This can be achieved through various feature extraction and selection techniques, such as principal component analysis (PCA), information gain, etc. Third, model training and optimization. The algorithm will select appropriate machine learning or deep learning models according to the requirements of personalized content, and train them in combination with large data sets. And during the training process, various optimization algorithms and techniques can be used to improve the performance and accuracy of the model. Finally, for the generation of personalized recommendation content, the algorithm will use a trained model to combine the user’s historical behavior, interests, and preferences to output and predict personalized content or recommendation results that match the user’s interests on the platform.
Example of predicting personalized content
Almost every social media platform is creating and using personalized recommendation services. Due to its occurrence behind the scenes, some customers may not be aware of its existence.
Here are some familiar examples:
Netflix
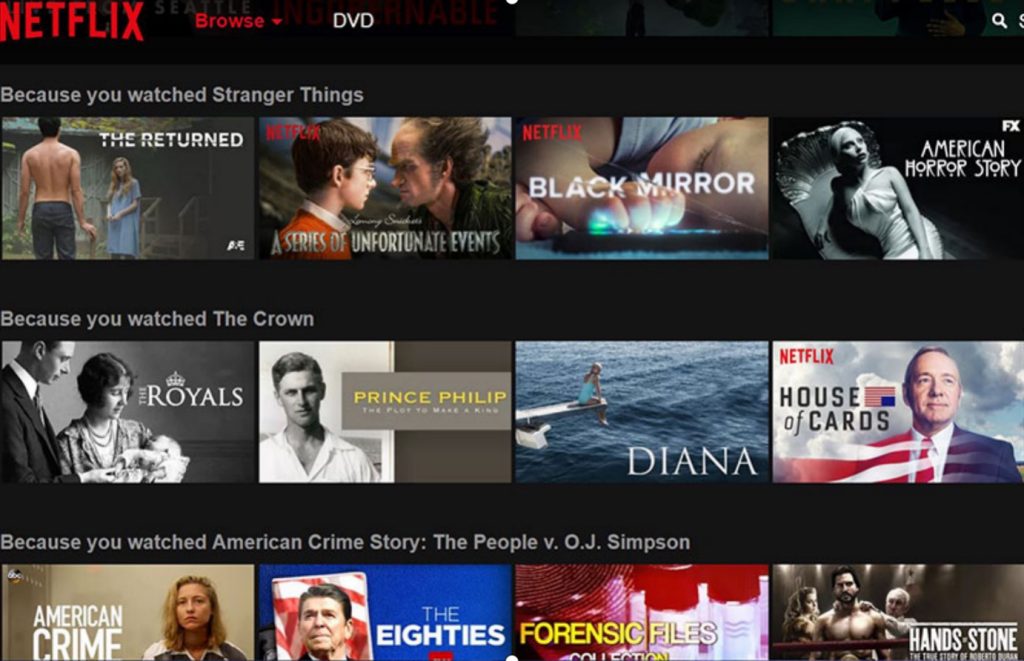
Netflix has over 57 million users worldwide. It is impossible to create a single homepage to meet their collective interests.On the contrary, it uses a complex set of algorithms to determine each person’s preferences. Then, their personal pages will be filled with movies and TV programs that suit them.
Thread
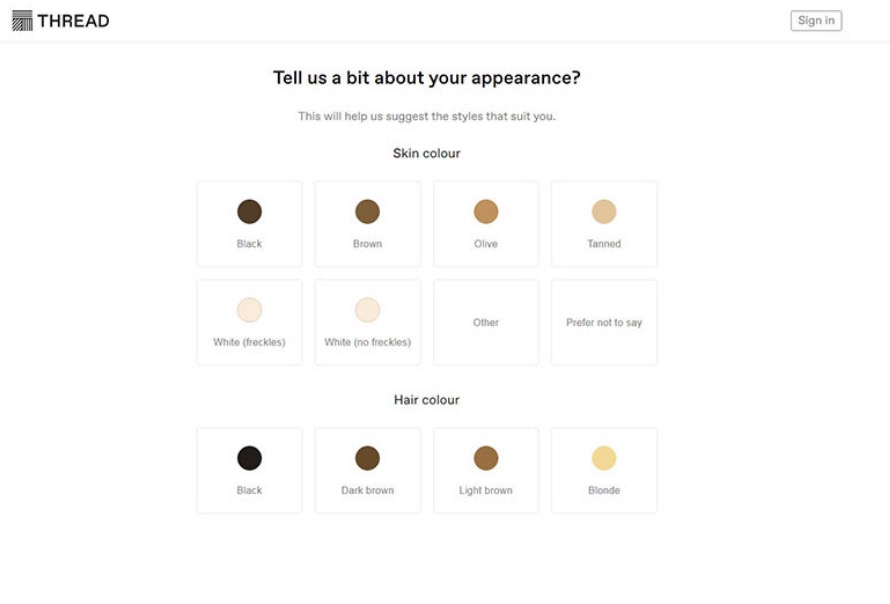
Clothing is a very personalized style expression. No company can reliably frame everyone’s style, and personalized content recommendation algorithms can approach individual aesthetics. Thread uses artificial intelligence to remember the preferences of each customer. Then it uses this mode to personally suggest clothing choices. The more data they collect, the closer their suggestions are to being accepted.
Disadvantages of personalized recommendation content
Privacy issue: Personalized push algorithms require the collection of a large amount of user data, which has the potential to infringe upon personal privacy and result in data abuse.
The reason why personalized recommendations may lead to user privacy leakage is that, as mentioned earlier, personalized recommendations require the collection and analysis of user personal data and behavioral information in order to provide them with customized recommendation content. These personal data and behavioral information may include sensitive information such as user browsing history, purchase history, geographic location, etc. If these data are not properly protected or abused, it will put users at risk of privacy leakage.
Error information and bias amplification: The algorithm will prioritize several ways in which the algorithm can continue error information by prioritizing participation rather than accuracy.
Firstly, algorithms can prioritize content that can generate high user engagement, such as likes, comments, and shares. This may lead to sensational or controversial content (including erroneous information) being amplified, as these contents typically generate more engagement than accurate information (Crawford, 2021). Secondly, algorithms may rely on user feedback signals (such as click through rates or dwell times) to determine the relevance and quality of content. If users are more likely to click or spend time on misleading or exaggerated content, algorithms may interpret it as a correlation signal and prioritize such content in future recommendations (Andrejevic, 2019). In addition, algorithms can use personalized data and user preferences to customize content recommendations. If the user has previously interacted with incorrect information or shown interest, even if the information is inaccurate, the algorithm may continue to recommend similar content to maintain user engagement.
The reduction of content diversity: Personalized recommendations often limit users’ access to different perspectives and content, leading to a narrowing of the information landscape.
Firstly, personalized algorithms will filter out content related to user interests for recommendations based on their historical behavior and preferences. This filtering process ignores content that is unrelated to the user’s interests or has different viewpoints, thereby limiting the user’s exposure to different viewpoints and content. Secondly, personalized algorithms also have the problem of filtering bubbles. The “foam” of information filtering allows users to only access content that meets their own interests, while ignoring other views and content (Andrejevic, 2019). This filtering bubble effect will further limit the opportunities for users to come into contact with different viewpoints, leading to a narrowing of the user information landscape.
“The environment in which a person only hears the viewpoints they agree with reinforces pre-existing beliefs and attitudes, and makes it difficult for the individual to understand and consider other viewpoints” (Sunstein 2017).
Echo chamber effect
A digital Echo chamber effect to an online environment or platform where individuals can only access information, viewpoints, and viewpoints that align with their existing beliefs and preferences. In a Echo chamber, individuals are less likely to encounter different viewpoints, challenging viewpoints, or conflicting information (Nguyen et al., 2014). This may lead to the strengthening of existing beliefs, biases, and ideologies, as well as limited understanding of complex issues. Echo chamber may lead to polarization, tribalism, and the spread of misinformation, as individuals have less exposure to alternative perspectives and critical analysis. The algorithms used in social media platforms and personalized recommendation systems play an important role in creating and continuing Echo chamber effect. These algorithms prioritize content that is more likely to attract users, forming a feedback loop that presents content that aligns with their preferences, reinforces their existing beliefs, and limits exposure to different viewpoints.
In fact, personalized recommendations can also lead to silos of information, causing users to repeatedly encounter similar ideas and opinions. For example, if a user likes to read science fiction, a personalized recommendation system might recommend more science fiction to that user, while ignoring other genres such as history and literature. In this way, users are trapped in information silos, only accessing content similar to their interests, and unable to gain broader knowledge and perspective.
Impact on society and discourse:
Such algorithms exacerbate the polarization of public discourse by personalizing outcomes and information selection. The selection of personalized algorithms based on the user’s personal characteristics, behaviors, social relationships and other factors leads to users only being exposed to information and opinions that conform to their own views, forming the so-called “filter bubble” or “information island” (Just&Latzer, 2016). This personalized selection makes users more inclined to accept and believe information that is consistent with their own views, while ignoring or rejecting information that contradicts them (A Society, Searching, 2018). This filter bubble effect contributes to the polarization of public discourse, making dialogue and understanding between different perspectives more difficult. Second, personalised algorithms undermine the foundations of democratic dialogue. The personalized results chosen by the algorithm only expose users to information that is consistent with their own views, ignoring other views and opinions (Pasquale, 2015). This personalized selection weakens dialogue and communication between users and different perspectives, making democratic dialogue more difficult. Finally, the choice of personalized recommendation algorithms may also lead to information asymmetry, making it easier for certain views and opinions to be amplified and disseminated, while others are marginalized and ignored. This information asymmetry further undermines the principles of equality and diversity in democratic dialogue.
Manipulation and control:
In fact, personalized recommendation algorithms can be abused by capital and power to manipulate and control users’ opinions and behaviors (Crawford, 2021). This means that personalized recommendation algorithms can shape user opinions and behaviors by filtering and excluding certain information, thereby influencing user choices and decisions. Personalized advice can have a negative impact on democracy, diminishing the diversity and autonomy of the public. In fact, by analyzing users’ interests and preferences, algorithms can selectively push specific information and content to users to achieve certain goals, such as political propaganda, public opinion guidance, etc. (Andrejevic, 2019). Such manipulation and control may weaken the public’s ability to think and judge independently, and affect public debate and opinion formation in a democratic system.
“We cannot deny the existence of the digital age, nor can we stop the progress of the digital age, just as we cannot resist the power of nature.”
——Negroponte’s Digital Survival
People have officially entered the era of big data, and how to better accept information in the context of big data is worth thinking about and improving.
Platform:
The platform needs to optimize its personalized recommendation algorithms and explore more ways to promote the production and recommendation of content with public value through algorithms. In terms of platform content production, algorithmic analysis can be used to gain insights into the common psychology of the public, providing more basis for media content production; In terms of content distribution, personalized algorithms can be used to promote content with public value to a wider audience. In addition, designers of personalized recommendations can take some measures, such as introducing diversity indicators to evaluate the diversity of recommended content, providing diverse recommended content, or displaying the diversity information of recommended content to users to help them better understand their information consumption tendencies and encourage users to engage with more content with different perspectives (Pasquale, 2015).
Media content:
The more information shared by people on social networks is often related to emotions, attitudes, and positions. However, the media still needs to adhere to the pursuit of truth and the principles of objectivity and calmness in news reporting. The media still needs to maintain a supply of content with public value, professional standards, and balanced aspects, otherwise no matter how algorithms and platforms are optimized, people will not be able to obtain a full and comprehensive understanding of the social environment.
In addition, the platform also has an inseparable regulatory responsibility for the published content. In order to maintain the quality of platform media content, the platform uses content review tools to identify suspicious posts and comments. For example, Reddit uses machine learning models to discover suspicious comments, which are further reviewed by human reviewers. Similarly, Facebook uses artificial intelligence tools to detect abuse and fraudulent behavior in posts, images, and videos, with human auditors intervening when needed.
Reference:
A society, searching. (2018). In Algorithms of Oppression (pp. 15–63). NYU Press. http://dx.doi.org/10.2307/j.ctt1pwt9w5.5
Andrejevic, M. (2019). Automated culture. In Automated Media (pp. 44–72). Routledge. http://dx.doi.org/10.4324/9780429242595-3
Crawford, K. (2021). The Atlas of AI: Power, politics, and the planetary costs of artificial intelligence. Yale University Press.
Gilmanov, A. (2023, February 9). Here’s why personalization algorithms are so efficient. TMS Outsource. https://tms-outsource.com/blog/posts/personalization-algorithms/
Just, N., & Latzer, M. (2016). Governance by algorithms: Reality construction by algorithmic selection on the Internet. Media, Culture & Society, 39(2), 238–258. https://doi.org/10.1177/0163443716643157
Musto, C., Semeraro, G., Lops, P., de Gemmis, M., & Narducci, F. (2012). Leveraging social media sources to generate personalized music playlists. In Lecture Notes in Business Information Processing (pp. 112–123). Springer Berlin Heidelberg. http://dx.doi.org/10.1007/978-3-642-32273-0_10
Nguyen, T. T., Hui, P.-M., Harper, F. M., Terveen, L., & Konstan, J. A. (2014, April 7). Exploring the filter bubble. Proceedings of the 23rd International Conference on World Wide Web. http://dx.doi.org/10.1145/2566486.2568012
Pasquale, F. (2015). The black box society: The secret algorithms that control money and information. Harvard University Press.
Be the first to comment