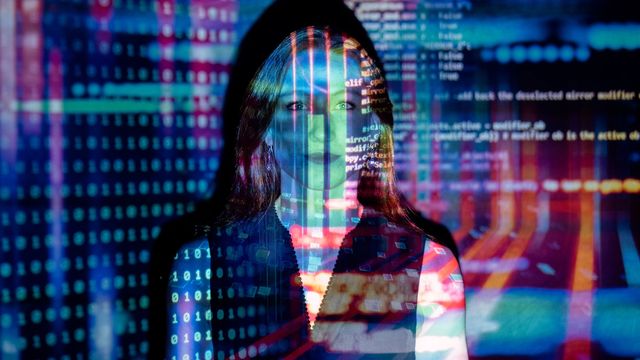
Introduction
Meta, with an audience of billions, a plethora of social media sites under its name, is arguably one of the most influential tech companies. However, before Meta, there was Facebook, and before that, there was Facesmash, the computer program that allowed users to rate whether women at Harvard University were ‘hot or not’. This example is not here to undermine the abilities of Mark Zuckerberg, who, it should be added, denies that Fashmash and Facebook have anything to do with each other (Zuckerberg Answers Question about Facemash, n.d.). However, it does illustrate the subjective histories of companies that are, in turn, bound to seemingly objective artificial intelligence (AI), machine learning (ML) and algorithms.
AI, ML and their algorithms did not appear from the abyss of objectivity; they are part of the subjective world and are informed by the histories, practices, and politics of living and breathing humans—in the case of Meta, a twenty-something sophomore. The histories of technology companies, the platforms like Facebook and Instagram and the content of the Internet inform our daily lives. They, through images, text and recommendation proliferate meaning. A study published by Professors Douglas Guilbeault and Solène Delecourt in 2024 demonstrates how online images, not text, continue to amplify gender bias leading to stereotypes. In examining the result of that study and reconsidering the approach to algorithms this article calls for change; firstly on how we view algorithms and secondly on how we approach the problem. To do so, it is essential to understand what algorithms are, then, ask how they become biased. Finally, we must consider what is being done and how we can resolve the problem further.
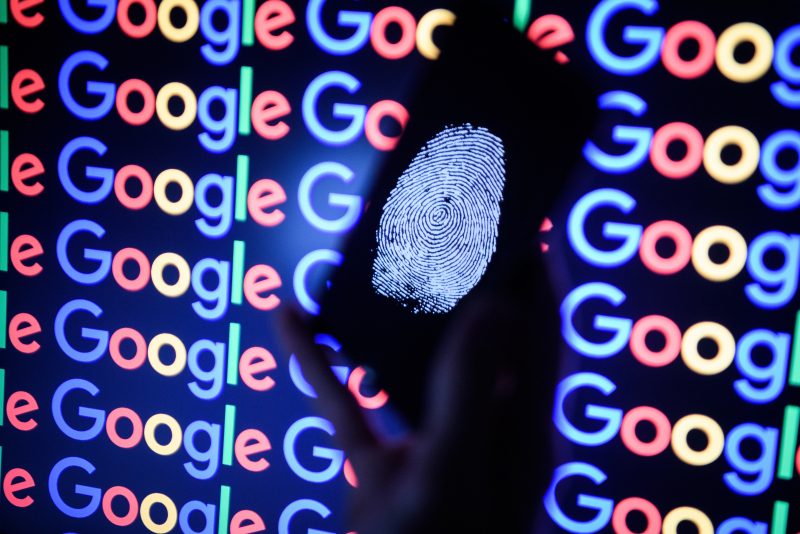
Algorithm bias
Algorithms, along with data and hardware, are part of AI and ML. They are automated instructions, the “rules and processes established for activities such as calculation, data processing and automated reasoning” (Flew, 2021, p. 83). Through automated assessments, algorithms assign relevance and classify different parts of the data. Classifying gives data order, separating and in organizing it into relevant groups, the classification creates hierarchies. The more data and greater engagement improves the algorithm’s decision-making. Idealistically speaking, this process provides the best possible rational action and exceeds human ability.
However, algorithms and AI are not producing objectified neutral results; they are highly biased, and this is not a new charge. For example, Amazon’s automated recruitment system trained to rate applicants by observing ten years of previous resumes “penalized resumes from female applicants with a lower rating” (Denison, 2023). Chatbot Tay, who learnt from others on the Internet, was shut down after 24 hours after becoming a misogynist and racist. The Correctional Offender Management Profiling for Alternative Sanctions aimed to predict which criminals would re-offend; in doing so, it misclassified black defendants as more likely to reoffend and labelled white defendants less of a risk (Denison, 2023). However, when we look at bias as an outcome, the guilt usually lies with the instructions, and “The result has been a focus on adjusting technical systems to produce greater quantitative parity across disparate groups” (Crawford, 2012, p.130). In short, there seems to be a misguided belief, the readjustment of algorithms will wrinkle out the gender, racial and ageist bias. However, as time passes, this approach seems redundant. Worse still, as “algorithms come to ‘influence…what we think’ about agenda setting and about framing, and ‘consequently how we act'” (Flew, 2021, p. 83), the problem seems obstreperous. We are now on board the metaphorical runaway train with billions of other people and the conductors have no clue where it’s headed.
Classification
To understand the production of bias within algorithms, we must first examine data tagging and classification. Data tagging assigns a label to data; this sets it apart and makes it easier to search for (Data Visibility and Security Solution, n.d.). If we take an image of a smiling black woman for example, it will be tagged with human, gender, race, it can also be assessed on age, young or old and emotion, happy, sad, angry. The multiple tags are then organised in a hierarchical structure, where the image falls in the hierarchy will determine where it appears in a search. Beyond the image these hierarchies can be used to assess someone’s viability for a job, security risk, credit, income or whether they are likely to reoffend.
Assigning relevance and classifying data are not new, nor do they solely belong to the objective. Stuart Hall, a prolific Cultural Studies figure, argues that classification is a “biological and genetic phenomena, which are a feature of how we are constituted as human beings” (Hall, 1997, p.10). We classify as a way to understand the world and the culture around us, it tells us what something is and isn’t and what it belongs to, allowing us to communicate effectively. Classifying objects, places, people etc is part of “practices that are involved in the production of meaning” (Hall, 1997, p.10). Importantly for Hall, and the biological amongst us, these meanings and classifications are not fixed. They shift and alter with temporality. However, there is an urge to fix meaning, to define class, race, gender with permanency. This, Hall argues, is how power intervenes to create stereotypes, it “create(s) a relationship between the image and a powerful definition of it to become naturalized” (Hall, 1997, p.19). Stereotypes can lead to bias, for example, if we consistently see images of women cooking, that is a stereotype and this can lead to bias about women’s roles in society. The fixing of such ideas reproduces social norms, something the church, state and education has done for centuries. The concern now is that the role is in the remit of algorithms.
There is a striking similarity between the biological tendency to classify and the objective classification of data tagging algorithms. Both are trying to organise as a way of understanding, give meaning and have the power to fix ideas all of which reinforce stereotypes and create bias. However, the idea that algorithms are objective is a diversion of the truth. They “have been constructed from a particular set of beliefs and perspective(s). The chief designers…are a small and homogenous group of people, based in a handful of cities, working in an industry that is currently the wealthiest in the world” (Crawford, 2021, p. 13). What the algorithms are demonstrating is not “neutral reflections of the world” (Crawford, 2021, p. 13) but the embedded histories and practices of the humans behind them and the culture they inhabit.
Gender bias in Google Images
As with the earlier example of Chatbot Tay, it would be fair to assume that algorithms which exhibit bias are tested, discontinued, and or reworked before they have any real effect. However, in January 2024, a study published by Professors Guilbeault and Delecourt demonstrated how online images, not text, continues to amplify gender bias. The study compared more than half a million images from Google and billions of words from Wikipedia and Internet Movie Database. Exploring word association with occupations like “nurse”, and “carpenter” and social roles such as “friend” and “caregiver”, the test retrieved the top one hundred images related to these words and then collected their frequency in the news. The results “found that female and male gender associations are more extreme among images retrieved on Google than within text from Google News” (Gilbert, 2024), specifically speaking, the bias towards men in images is four times stronger. To put it another way, Google Images serves the idea that most humans are men. Women are not only “underrepresented in images, compared with texts” (Guilbeault et al., 2024, p.1050), but also misrepresented in occupation. When the researchers cross referenced the findings with the U.S. Bureau of Labor Statistics “they found that gender disparities were much less pronounced than in those reflected in Google images” (Gilbert, 2024). Finally, the research showed that “being exposed to gender bias imagery led to stronger bias in people’s self-reported beliefs about the gender of occupations” (Guilbeault et al., 2024, p.1054). This is an example of the algorithm bias playing out through the images Google serves. Those images have a social impact, they reinforce harmful gender stereotypes that not only under and misrepresent women but reproduce bias within people’s beliefs.
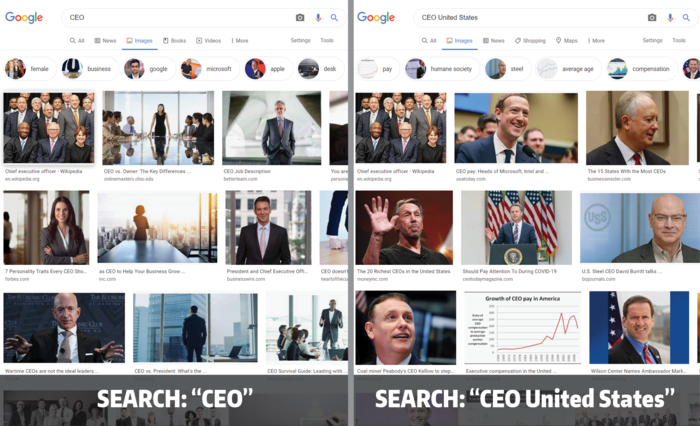
Action on harmful images
A report on gender stereotypes in the U.K. by the Advertising Standards Authority (ASA) and the Committee of Advertising Practice (CAP) found that “wherever they appear or are reinforced, gender stereotypes can lead to mental, physical and social harm which can limit the potential of groups and individuals” (Depictions, Perceptions and Harm a Report on Gender Stereotypes in Advertising, 2019, P.37). This can result in “suboptimal outcomes for individuals and groups in terms of their professional attainment and personal development” (Depictions, Perceptions and Harm a Report on Gender Stereotypes in Advertising Summary Report, 2019, p.9.). Aligning with the UK Equality Act 2010, which prohibits discrimination of protected characteristics, including age, disability, sex and sexual orientation, the ASA placed a ban on gender stereotypes in its advertising. Specifically speaking, the Code of Broadcast Advertising (BCAP) rules that “Advertisements must not include gender stereotypes that are likely to cause harm, or serious or widespread offence” (ASA, n.d.). The action taken by the ASA and CAP provides a progressive approach to gender stereotypes. However, it is arguably easier to implement this law because on the other side of an advertising stereotype is an advertising agency who can be held accountable. It is proving harder to implement such ideas when algorithms are involved. As defined earlier, subjective histories and perspectives are at the heart of platforms and tech companies and their algorithms are affecting billions of people. So if we have proof that Google Images are in fact biased, misrepresenting and under-representing women. If these biases create stereotypes that are harmful, then it is surely time to hold those responsible accountable for the subjective suffering. Ultimately, whether the algorithms intended it or not, harm is harm.
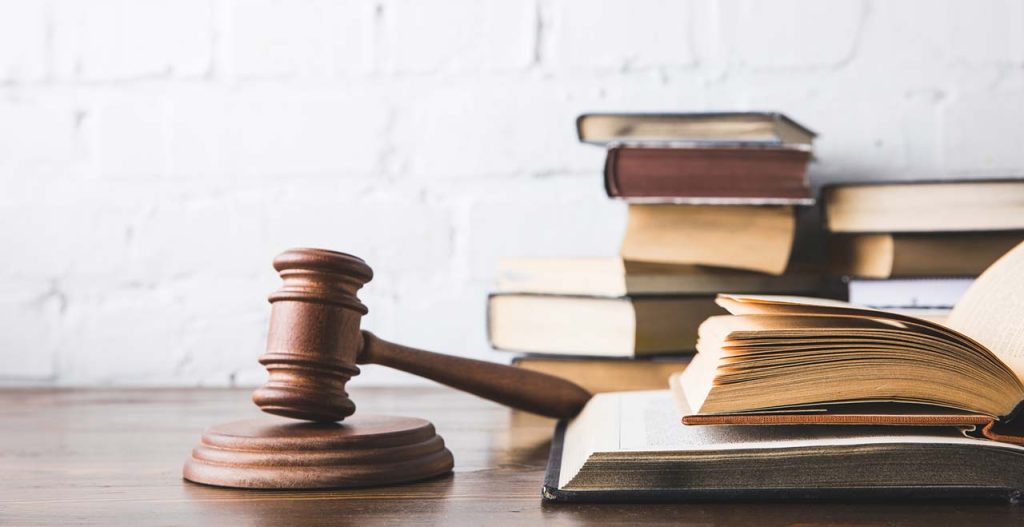
What can be done in Australia?
In Australia, as with the UK, it is unlawful to discriminate based on several protected attributes (Australian Government, 2022). Turning to the Sex Discrimination Act 1984, section 5B, regarding discrimination on the grounds of gender identity, a person is discriminated against if they are treated less favourably than if they have a different gender identity (Sex Discrimination Act 1984, 2021). The article published by Professors Guilbeault and Delecourt demonstrates how Google serves biased images that discriminate against women simply because of their gender. It could be argued that this is ‘indirect discrimination’. What then needs to be taken into account is the nature and extent of the resulting disadvantage and the feasibility of overcoming or mitigating that disadvantage. As the article proved through national statistical data, the conditions Google Images produces are not reasonable as they do not reflect the reality outside of the search engine. Furthermore, the ASA and CAP show the resulting disadvantage of such imagery is hard to overcome as it stunts professional attainment and personal development. When images seen by billions are fundamentally re-enforcing bias, which in turn informs our norms, the disadvantage can neither be mitigated nor overcome. Therefore it is important to hold the companies accountable for the algorithms that are reproducing blanketed discrimination.
Conclusion
This article calls for the reconsideration of algorithms; rather than automated instructions that form isolated objective outcomes, they are, in fact, implemented by humans and are embedded with subjective histories and practices. Their processes of classification and hierarchical structures are not isolated to technologies but are part of the biological human condition. As a consequence, algorithms are producing biased, sexist, ageist, and racist results, which in turn is causing harm and affecting the lives of billions of people every day. As demonstrated by Professors Guilbeault and Delecourtand, there is a proven gender bias against women within Google’s algorithms leading to the reproduction of stereotypes. As seen in the UK, it is possible to challenge stereotypes and the harm they cause. Australian Sex Discrimination Act 1984 outlines that discrimination occurs if a person is treated less favourably than if they have a different gender identity, therefore, algorithms and their subsequent bias should not go unchallenged. No longer can a laissez-faire approach be sufficient for something with the potential to produce meaning that affects billions of people. It is time to stop the metaphorical train and demand to know where we are going.
References
Advertising Standards Authority | Committee of Advertising. (n.d.). 04 Harm and offence. Www.asa.org.uk. Retrieved April 5, 2024, from https://www.asa.org.uk/type/broadcast/code_section/04.html#:~:text=4.14
Australian Government. (2022). Australia’s anti-discrimination law. Attorney-General’s Department. https://www.ag.gov.au/rights-and-protections/human-rights-and-anti-discrimination/australias-anti-discrimination-law
Crawford , K. (2021). Classification. In The Atlas of AI: Power, Politics, and the Planetary Costs of Artificial Intelligence (pp. 123–149). Yale University Press.
Crawford, K. (2021). Introduction. In The Atlas of AI (pp. 1–21). Yale University Press. https://doi.org/10.2307/j.ctv1ghv45t.3
Data visibility and security solution by ManageEngine DataSecurityPlus. (n.d.). ManageEngine DataSecurityPlus. Retrieved April 4, 2024, from https://www.manageengine.com/data-security/what-is/data-tagging.html
Depictions, Perceptions and Harm A report on gender stereotypes in advertising. (2019). https://www.asa.org.uk/static/uploaded/e06425f9-2f9f-44c3-ae6ebd5902686d44.pdf
Depictions, Perceptions and Harm A report on gender stereotypes in advertising Summary report. (2019). https://www.asa.org.uk/static/uploaded/e27718e5-7385-4f25-9f07b16070518574.pdf
Flew, T. (2021). Regulating Platforms. In Perlego (1st ed.). Polity. https://www.perlego.com/book/3118806/regulating-platforms-pdf
Gelashvili, T. (2022, June 22). Skrastins & Dzenis Successful for PNB Banka in LCIA Arbitration. CEE Legal Matters. https://ceelegalmatters.com/latvia/20320-skrastins-dzenis-successful-for-pnb-banka-in-lcia-arbitration
Gilbert, K. (2024, February 14). Online images may be turning back the clock on gender bias, research finds. Haas News | Berkeley Haas. https://newsroom.haas.berkeley.edu/research/internet-images-may-be-turning-back-the-clock-on-gender-bias-research-finds/
GOV.UK. (2010). Equality Act 2010. Legislation.gov.uk. https://www.legislation.gov.uk/ukpga/2010/15/part/2/chapter/2/crossheading/discrimination
Guilbeault, D., Delecourt, S., Hull, T., Desikan, B. S., Chu, M., & Nadler, E. (2024). Online images amplify gender bias. Nature, 1–7. https://doi.org/10.1038/s41586-024-07068-x
McQuate, S. (n.d.). Google’s “CEO” image search gender bias hasn’t really been fixed: study. Techxplore.com; University of Washington. Retrieved April 18, 2024, from https://techxplore.com/news/2022-02-google-ceo-image-gender-bias.html
Megorskaya, O. (2023). Council Post: Training Data: The Overlooked Problem Of Modern AI. Forbes. https://www.forbes.com/sites/forbestechcouncil/2022/06/27/training-data-the-overlooked-problem-of-modern-ai/?sh=499ca4e3218b#:~:text=You%20need%20to%20be%20able
Neal, L. (n.d.). Google will be eager to avoid the sort of drawn-out battle and fines that dogged Microsoft for the best part of a decade [Photograph ]. In Getty Images. https://www.politico.eu/article/google-propose-search-changes-to-eu-antitrust-regulators/
Sex Discrimination Act 1984, 5B (2021). https://www.legislation.gov.au/C2004A02868/2021-09-11/text
Sex Discrimination Act 1984, 7B (2021). https://www.legislation.gov.au/C2004A02868/2021-09-11/text
Stacy, D. (2023, August 31). Topic: Meta Platforms. Statista. https://www.statista.com/topics/9038/meta-platforms/#topicOverview
ThisIsEngineering, & Pexels. (n.d.). Code Projected Over Woman. https://www.pexels.com/photo/code-projected-over-woman-3861969/
United kingdom committee of advertising practice rules against gender stereotyping spotlight on public policy key features of rules. (2020). in weps. https://www.weps.org/sites/default/files/2020-12/CaseStudy_UnitedKingdom_Final.pdf
What is image classification? Basics you need to know | SuperAnnotate. (2023). Www.superannotate.com. https://www.superannotate.com/blog/image-classification-basics
Zuckerberg Answers Question About Facemash. (n.d.). Www.youtube.com. Retrieved April 11, 2024, from https://www.youtube.com/watch?v=BEsARBz8Cv4
Be the first to comment